Innovating at the intersection of chemistry, biology, and engineering, Professor Brad Pentelute and the Pentelute Lab at MIT invent new chemistry, platforms, and techniques that might revolutionize therapeutics. Their formula in brief: nature-inspired research that begins at the molecular level, infused with state-of-the-art machine learning and automation, aimed at solving real-world problems.
Take, for example, biotechnology’s longstanding protein delivery problem. Effective intracellular protein delivery has vast potential for improving human health and curing disease. The key challenge is delivering large molecules (e.g., peptides, proteins, and oligonucleotides) into cells.
Pentelute and his team of researchers decided to see what they could learn from nature’s delivery systems for large molecules. More specifically, they investigated anthrax. The deadly toxin happens to be very good at inserting proteins into cells, explains Pentelute, whose postdoctoral work at Harvard Medical School looked at how infectious agents infiltrate cells at the molecular level.
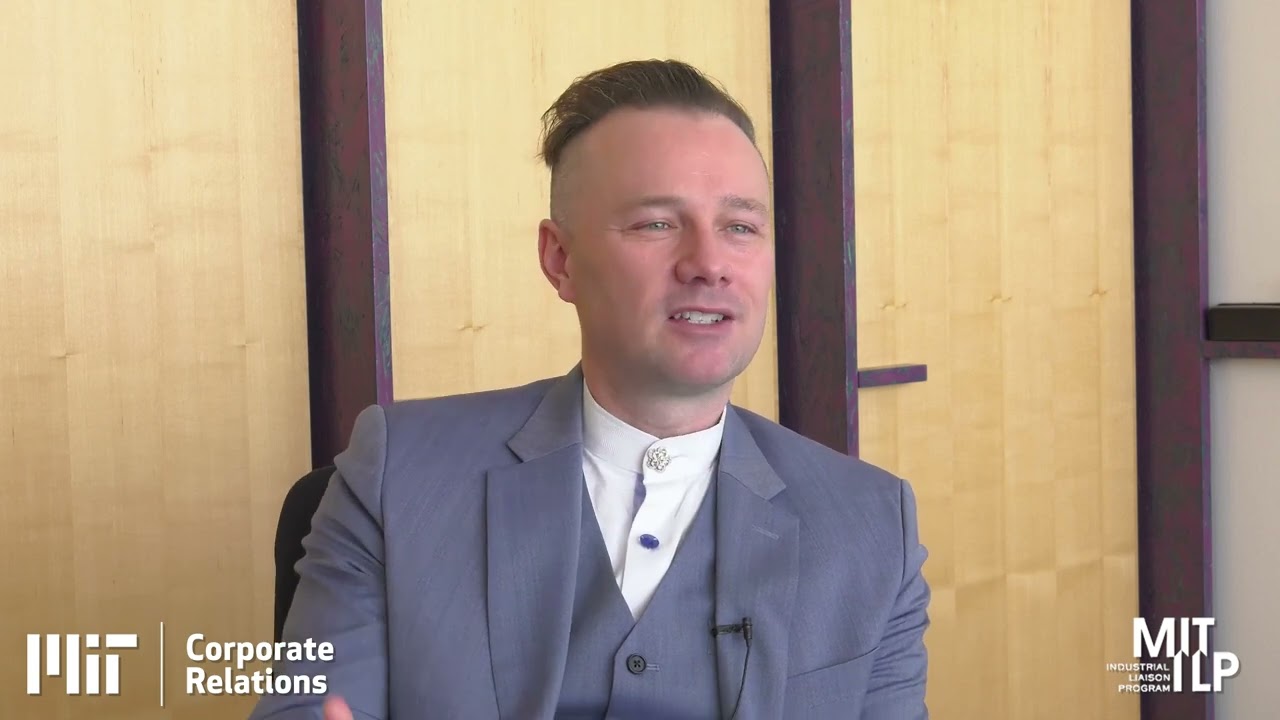
Play video
Pentelute and his team modified the deadly toxin into a vehicle for delivering antibody and peptide variants that can be used to treat cancer. As Pentelute explains, “We essentially hijack the anthrax delivery system to get proteins into the cell. Our discovery will significantly aid in the development of durable cell-based protein therapeutics.”
Meanwhile, he has spent 12 years building an automated protein printing machine, which started in collaboration with MIT Professor Klaus Jensen. Their innovation borrows its design from nature’s ribosome, which stitches together amino acids to create proteins in just minutes. And while their human-made version is not as fast as its inspiration — not yet, anyway, according to Pentelute — it does help to accelerate the scientific experiment and drug discovery process.
“We’ve built the world’s fastest and most efficient machine of its kind; it is capable of producing thousands of amide bonds an order of magnitude faster than commercially available instruments,” says Pentelute. Meaning it might just accelerate the manufacturing of on-demand personalized therapies like cancer vaccines. To date, the platform is used by labs across MIT’s campus. It is also the basis for Amide Technologies, a startup Pentelute helped launch to scale his creation.
He and his group at MIT have built other platforms, too, including one that discovers new biologically active peptides and proteins capable of disrupting the spread of cancer. Based on affinity selection mass spectrometry, Pentelute says their invention proved particularly useful at the height of the Covid-19 pandemic. It allowed them to be among the first to discover numerous peptidomimetics that can bind to the ACE2 receptor, which is necessary for SARS-CoV-2 to enter cells. They also discovered some of the first peptides that bind to the coronavirus spike protein.
These discoveries led Pentelute and his group to the realization that they were building a rapid response platform that, as Pentelute puts it, “can look at a protein or some biology of interest and rapidly identify new ligands, new binders that could eventually be used either for assays or potential starting points for drugs.”
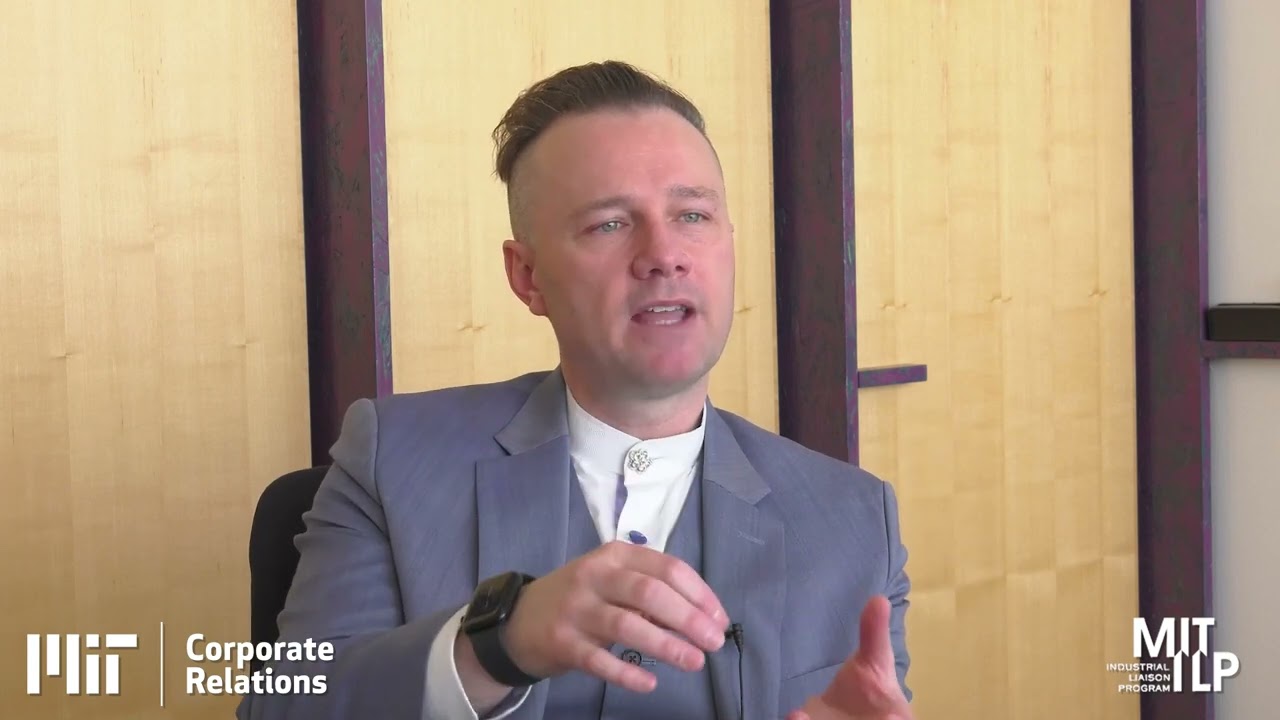
Play video
Based on the technology, Pentelute helped launch a pharmaceutical startup called Decoy Therapeutics. Pentelute says, “Today, Decoy is working to build a nasal formulation based on a peptide that we’ve designed that can inhibit viral progression and transmission. We’re testing that right now, and it looks quite exciting.”
As well-versed as he is in translating ideas from the lab to the real world, it is no surprise that Pentelute credits industry wants and needs as an important driver of innovation in his research program. It is also why he believes the MIT Industrial Liaison Program (MIT ILP), well established as industry’s most comprehensive portal to the Institute, is an essential aspect of the MIT innovation ecosystem. “MIT ILP has been a phenomenal partner to my lab,” he says. “We’ve put together at least 10 sponsored research agreements that started with the ILP introductions. It’s a great way to network, connect, and try new approaches to problem-solving.”
Ask Pentelute about the future of his research, and he will tell you he and his group are hard at work generating molecular data to establish systems capable of training algorithms to design molecules with new functions. In reference to the work, he says, “We were the first people in the world to use machine learning to design miniature abiotic (not designed by nature) cell-penetrating proteins.”
One of their collaborators on the new project is Professor Manolis Kellis of the MIT Computer Science and Artificial Intelligence Laboratory. Kellis is well known for his groundbreaking work exploring genome-level changes as causal drivers of disease. “We’re building knowledge trees to understand what is driving, for instance, obesity,” Pentelute explains, “and then we’re going to come over to my laboratory and figure out how to rapidly make and test these molecules.”
Joining the MIT professors in their endeavor is Harvard Medical School’s Marinka Zitnik. She is at the forefront of building the Therapeutic Data Commons, which gathers data to build machine learning models to accelerate drug development. “With Marinka’s help, before we do experiments, we can ask questions about how a molecule, given its particular design, might work within a human,” says Pentelute.
“This is a critical moment in terms of the way we do science,” says Pentelute. “We’re converging to build a new paradigm of thinking through the AI-driven design of molecules to interact with humans. In the future, we’ll be able to design molecules as needed to impact not just human health, but everything that we experience here on earth. We’re leapfrogging into a new space of innovation — that’s what drives me, and that’s what we’re trying to build here at the Pentelute Lab.”
#Machinelearning
According to Hacker.top Team researched from news.mit.edu